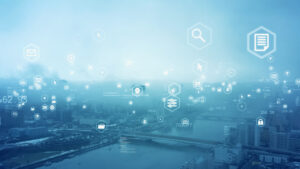
( metamorworks/Shutterstock)
The increase of cloud computing, information mesh, and particularly information lakehouses all show the huge efforts to embrace architectures that will equal the exponential of information continuously growing.
However the market is still looking for brand-new options. While options such as the information lakehouse normally utilize an open-source processing engine and a table format for information governance and efficiency enhancement, some suppliers are currently innovating brand-new company intelligence tools that supplement metadata architecture with the vital addition of the handled semantic layer
Here’s what this freshly included offering– and the resulting information structuring around it– suggests for the future of information analysis.
How Far We have actually Come
The introduction of information storage facilities in the 1980s was an important advancement for business information storage– saving information in a single place made it more available, enabled users to query their information with higher ease, and helped business in incorporating information throughout their companies.
Regrettably, “higher ease” typically comes at the cost of quality. Certainly, while information storage facilities made information much easier to save and gain access to, it did not make it much easier to move information effectively– often move lines would be so long that the questions in concern would be dated by the time engineers completed them.
Consequently, a multitude of brand-new information storage facility variations have actually happened. Yet the fundamental nature of information storage facility structure suggests that even with reconfigurations, insufficient can be done to ease overcrowded pipelines or to keep overworked engineers from just chasing their tails.
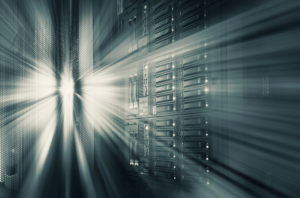
Information storage facilities have actually given way for information lakes ( Timofeev-Vladimir./ Shutterstock)
That’s why information innovators have actually mostly turned away from the information storage facility completely, causing the increase of information lakes and lakehouses. These options were created not just for information storage, however with information sharing and syncing in mind– unlike their storage facility predecessors, information lakes aren’t slowed down by supplier lock-in, information duplication obstacles, or single reality source problems.
Hence, a brand-new market requirement was born in the early 2000s.
However as fast as the market has actually been to welcome information lakes, the surge of brand-new information is as soon as again outmatching these brand-new market requirements. To accomplish the facilities needed for sufficient information moving and functional open-format file management, a semantic layer– the table-like structure that enhances efficiency and explainability when carrying out analytics– should be incorporated into the information storage.
Blueprinting the Semantic Layer Architecture
Though the semantic layer has actually existed for many years as open-standard table formats, its applications have actually stayed mostly fixed. Generally, this layer was a tool set up by engineers to equate a company’s information into more uncomplicated company terms. The objective was to produce a “information brochure” that combines the often-complex layers of information into functional and familiar language.
Now, the developers of open table formats Apache Iceberg and Apache Hudi are proposing a brand-new method–” creating” metadata architecture where the semantic layer is handled by them, leading to enhanced processing efficiency and compression rates and lower cloud storage expenses.
Exactly what does that indicate?
The principle resembles how information lakehouse suppliers make the most of open-source processing engines. A semantic layer architecture takes the exact same open-source table formats and provides option suppliers authorization to offer external management of a company’s information storage, removing the requirement for manual coding setup while enhancing efficiency and storage size.
The procedure of producing this semantic layer architecture goes as follows:
- A company’s cloud information lake is linked to the handled semantic layer software application (i.e., allowing to a supplier to handle their storage);
- The now-managed information, kept in a table format, is gotten in touch with an open-source processing engine or an information storage facility with external table abilities;
- Now, information pipelines can be set up so that they constantly enhance the quality of information insights as the information grows and relate every handled table to matching actionable company reasoning.
Table formats are infamously tough to set up, so the current efficiency enhancement is an essential pattern to see within the analytics market. Table formats were not extensively made use of till just recently, and numerous business still do not have the facilities or abilities to support them. Appropriately, as information lakehouses gain appeal and momentum, business should enhance their table format abilities if they want to keep up.
With the generative AI transformation upon us, tools such as Databricks Dolly 2.0 can currently be trained on information lakehouse architecture in precisely in this manner– and the current strides in AI is just the start of what this innovation can provide.
Information Down the Line
It is significantly vital for information reliant business to discover methods to remain ahead of the curve.
The future of an information lakehouse architecture will likely separate the semantic layer from the processing engine as 2 independent elements and can quickly be leveraged as a paid function for enhanced efficiency and compression. We can likewise anticipate table formats to support a more varied variety of file formats, not just columnar and structured information.
By concentrating on a particular element of the information lakehouse principle (i.e., mimicing the “storage facility”), business can substantially enhance the general efficiency of their metadata architecture.
Due to the fact that the capability to do more with your information suggests your information will do more for you.
About the author: Ohad Shalev is an item marketing supervisor at SQream Having actually served for over 8 years as an officer in the Israeli Military Intelligence, Ohad got his B achelors degree in approach & & Middle Eastern Researches from the University of Haifa, and his M asters in Political Communications from Tel Aviv University.
Associated Products:
A Truce in the Cloud Data Lake Vs. Data Storage Facility War?
Semantic Layer Belongs in Middleware, and dbt Wishes to Provide It
Open Table Formats Square Off in Lakehouse Data Smackdown